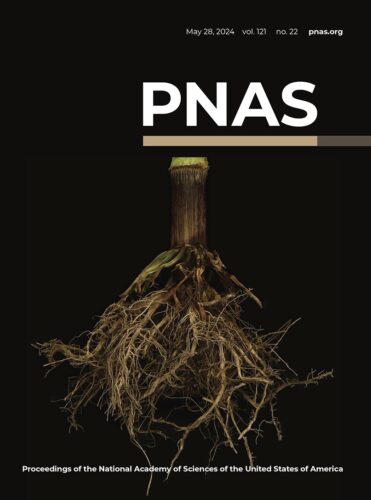
With scientific integrity and responsibility in mind, the National Academy of Sciences, the Annenberg Public Policy Center of the University of Pennsylvania, and the Annenberg Foundation Trust at Sunnylands recently convened an interdisciplinary panel of experts with experience in academia, industry, and government to explore rising challenges posed by the use of AI in research and to chart a path forward for the scientific community. The panel included experts in behavioral and social sciences, ethics, biology, physics, chemistry, mathematics, and computer science, as well as leaders in higher education, law, governance, and science publishing and communication. Discussions were informed by commissioned papers detailing the development and current state of AI technologies; the potential effects of AI advances on equality, justice, and research ethics; emerging governance issues; and lessons that can be learned from past instances where the scientific community addressed new technologies with significant societal implications.
Generative AI systems are constructed with computational procedures that learn from large bodies of human-authored and curated text, imagery, and analyses, including expansive collections of scientific literature. The systems are used to perform multiple operations, such as problem-solving, data analysis, interpretation of textual and visual content, and the generation of text, images, and other forms of data. In response to prompts and other directives, the systems can provide users with coherent text, compelling imagery, and analyses, while also possessing the capability to generate novel syntheses and ideas that push the expected boundaries of automated content creation.
Authors
- Wolfgang Blau
- Vinton G. Cerf
- Juan Enriquez
- Joseph S. Francisco
- Urs Gasser
- Mary L. Gray
- Mark Greaves
- Barbara J. Grosz
- Kathleen Hall Jamieson
- Gerald H. Haug
- John L. Hennessy
- Eric Horvitz
- David I. Kaiser
- Alex John London
- Robin Lovell-Badge
- Marcia K. McNutt
- Martha Minow
- Tom M. Mitchell
- Susan Ness
- Shobita Parthasarathy
- Saul Perlmutter
- William H. Press
- Jeannette M. Wing
- Michael Witherell